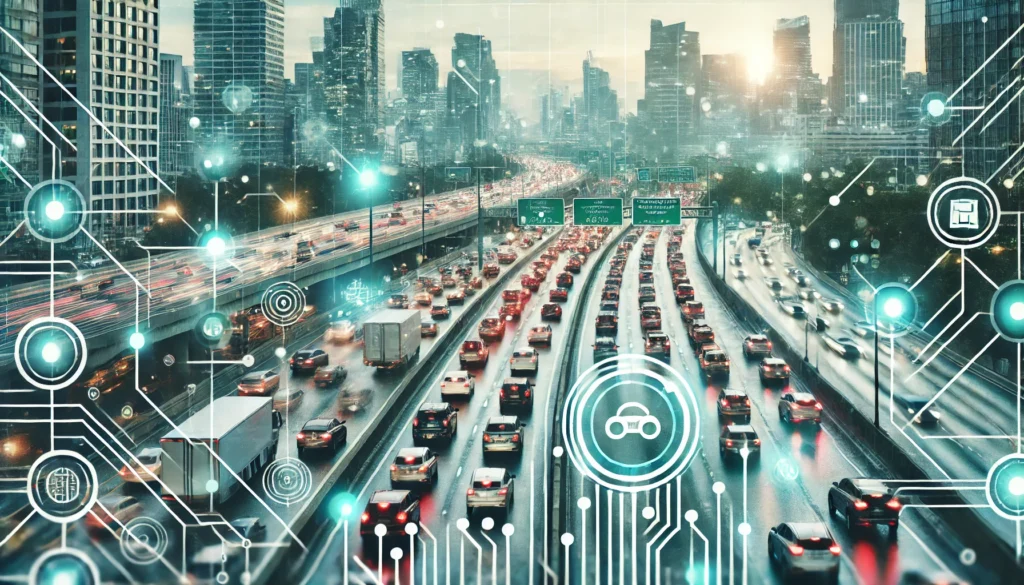
American cities face a daily struggle with traffic congestion. Whether it’s commuters packed on the highways during rush hour or delivery trucks maneuvering through busy downtown streets, traffic flow remains a critical challenge. For decades, city planners and companies have searched for ways to smooth traffic and reduce gridlock. Surprisingly, one of the most effective tools in this fight is data annotation.
Data annotation might sound like an abstract concept disconnected from the practical world of roads and stoplights. Yet, it’s the unseen force behind many of the advanced traffic management solutions we see today. By labeling and categorizing data collected from sensors, cameras, and GPS systems, companies can train AI models to analyze traffic patterns, predict congestion, and recommend real-time solutions. In essence, data annotation transforms raw traffic data into actionable insights.
Much like sorting through a stack of documents to find relevant information, data annotation sifts through the chaos of daily traffic data to provide clear guidance. It’s this groundwork that makes smarter, AI-driven traffic systems possible, helping cities and companies tackle the complex puzzle of urban mobility.
How Data Annotation Powers Traffic Management Systems
Traffic management systems rely heavily on data: video feeds from street cameras, GPS data from vehicles, signals from smart traffic lights, and even social media reports. However, all this data is useless without structure and meaning. This is where data annotation steps in.
Data annotation involves labeling elements in videos or images, like cars, buses, pedestrians, and traffic signs. For example, annotators might tag the position and speed of vehicles captured by road cameras. These labeled data sets then become training material for AI models, allowing them to understand and predict traffic patterns. A system trained with high-quality annotated data can analyze live feeds to detect accidents, monitor traffic density, and adjust traffic lights accordingly.
Consider this: an AI model fed with thousands of hours of annotated video can learn to distinguish between a slow-moving traffic jam and a minor traffic buildup that will soon clear. This difference is crucial for traffic management systems, as it allows them to take more accurate, context-driven actions, such as rerouting vehicles, adjusting traffic signals, or sending real-time alerts to drivers. Without the precise work of data annotation, the AI would be like a driver with a blindfold – full of potential but unable to navigate the road ahead.
Easing Urban Congestion with Real-Time Data Annotation
Traffic congestion is a dynamic problem, constantly changing throughout the day. To effectively manage it, companies need to react in real time. This is where real-time data annotation becomes invaluable. Systems equipped with AI models trained on annotated data can analyze live traffic feeds and respond instantaneously.
For example, some cities in the USA have implemented adaptive traffic signals that use real-time data to optimize the flow of vehicles. These signals receive input from sensors and cameras at intersections. The raw data, annotated and processed by AI, enables the system to identify traffic build-up and adjust the timing of lights to keep cars moving efficiently. This flexibility prevents minor congestion from escalating into full-blown gridlock, reducing travel times and improving overall traffic flow.
The beauty of this approach lies in its adaptability. Because the AI is constantly fed annotated data from real-world conditions, it can adapt to changes like road closures, accidents, or seasonal traffic variations. The ability to interpret and act on real-time data makes data annotation a cornerstone of modern traffic management.
Improving Traffic Predictions with Historical Data Annotation
Managing traffic flow isn’t just about responding to real-time conditions; it also requires predicting future congestion. Historical traffic data, when annotated correctly, provides a treasure trove of insights for making accurate predictions.
Companies analyze years of historical data that have been meticulously labeled to train AI models. This data might include information like traffic density at different times of the day, the impact of weather conditions on traffic patterns, or the influence of local events on congestion. By feeding annotated historical data into machine learning models, these systems learn to recognize patterns and make forecasts.
Imagine a logistics company using an AI-powered system to plan delivery routes. The system, trained on annotated data, can predict high-traffic areas and times, suggesting optimal delivery windows and routes to avoid delays. This capability isn’t limited to delivery services; ride-sharing companies, public transportation, and emergency services can all leverage these predictions to improve operations and reduce congestion.
The Human Element: Annotators Behind the Traffic Data
Despite the advances in AI and automation, human judgment is still at the heart of data annotation. Annotators meticulously label traffic data, categorizing vehicles, identifying congestion points, and tagging road features. This work requires attention to detail and a deep understanding of context – recognizing the difference between a stalled vehicle and normal stop-and-go traffic, for example.
In cities where traffic patterns are heavily influenced by cultural nuances or unique driving behaviors, local annotators bring invaluable context to the data. They help ensure that AI models are trained with culturally relevant examples, making the systems more effective in diverse environments.
Much like city planners rely on a team of experts to design urban infrastructure, AI systems depend on annotators to lay the foundation for their learning. The quality of this annotation work directly impacts how well AI models can interpret and manage traffic data.
Challenges of Annotating Traffic Data
Annotating traffic data is not without its challenges. One of the primary difficulties is the sheer volume of data involved. Cities generate an enormous amount of information every minute – from surveillance cameras, GPS tracking, and sensors embedded in roads. Each frame of a traffic video might contain dozens of vehicles, pedestrians, cyclists, and road signs, all of which need to be accurately labeled for the AI to learn effectively.
Additionally, traffic scenes are highly dynamic. Lighting conditions change throughout the day, weather can obscure visibility, and unexpected events, like accidents or construction, alter typical traffic flows. Annotating this data requires a nuanced approach, as annotators must differentiate between normal traffic variations and events that could significantly impact flow.
Despite these challenges, the work of annotators remains indispensable. AI models built on rigorously annotated data are far more reliable, capable of adapting to a wide range of conditions and providing accurate, actionable insights.
The Future: Smarter Cities with Better-Managed Traffic
The benefits of data annotation for traffic management are already being realized, but the future holds even greater potential. As cities invest in smart infrastructure, they’ll rely on increasingly sophisticated AI models trained on vast, high-quality annotated datasets. These systems will integrate data from various sources – not just cameras and sensors but also crowdsourced information from smartphones, public transit systems, and even social media.
In the future, we might see fully automated traffic management systems that can predict and prevent congestion before it occurs. These systems will be able to monitor traffic in real time, predict future conditions based on historical patterns, and make autonomous decisions to redirect vehicles, adjust traffic signals, or deploy public transportation resources as needed.
Data annotation is the key to this vision. By continually feeding AI with high-quality annotated data, cities can build smarter, more responsive traffic systems that adapt to changing conditions and keep traffic flowing smoothly. It’s a future where the invisible work of annotators today paves the way for safer, more efficient roads tomorrow.
Conclusion
Data annotation is the unsung hero of modern traffic management in American cities. It transforms raw data into structured insights that power AI-driven systems, improving traffic flow, reducing congestion, and enhancing urban mobility. Whether it’s real-time responses to changing conditions or forecasting future traffic patterns, annotated data is at the core of these advancements.
While the work of data annotation may be behind the scenes, its impact is felt on city streets every day. From adaptive traffic signals to predictive routing for delivery services, the results are clear: smoother traffic, shorter commutes, and less gridlock. As technology continues to evolve, data annotation will remain a vital component in building smarter, more connected cities.
FAQs
- What is data annotation in traffic management?
Data annotation in traffic management involves labeling data from various sources like cameras, sensors, and GPS to train AI models. These labels help the AI interpret traffic patterns, identify congestion, and make real-time adjustments. - How does data annotation improve traffic flow?
Annotated data allows AI models to understand traffic conditions, predict congestion, and adjust traffic signals or suggest alternative routes, thereby improving traffic flow and reducing gridlock. - Can data annotation help with real-time traffic management?
Yes, annotated real-time data allows AI systems to monitor traffic conditions as they happen, enabling dynamic responses such as adjusting signal timings or rerouting vehicles to avoid congestion. - Why is human judgment necessary for traffic data annotation?
Humans bring context and nuanced understanding to data annotation. They can distinguish between normal traffic patterns and anomalies, ensuring AI models are trained with accurate, relevant information. - What challenges are involved in annotating traffic data?
Challenges include the large volume of data, the need for precise labeling in dynamic and varied conditions (like changing weather or lighting), and the requirement to differentiate between subtle traffic variations. - What is the future of traffic management with data annotation?
The future points toward fully integrated, AI-driven traffic systems capable of real-time monitoring, prediction, and autonomous management of urban traffic, all built on the foundation of high-quality annotated data.